Clinical prediction for surgical versus nonsurgical interventions in patients with vertebral osteomyelitis and discitis
Highlight box
Key findings
• The top ten XGBoost model clinical features predicting vertebral osteomyelitis and discitis (VOD) outcomes: admit diagnosis of fever, negative culture, Staphylococcus aureus culture, PaO2:FiO2, admit diagnosis of intraspinal abscess and granuloma, admit diagnosis of sepsis, race, troponin I, acid-fast bacillus culture, and A-a gradient.
What is known and what is new?
• VOD has significant morbidity and mortality, there is no reported clinical support tool to predict the likelihood of benefit from a surgical intervention (during the same hospital stay).
• This manuscript reports a machine learning-based clinical support tool to predict the indication for surgical intervention (during the same hospital stay) by analyzing 50 clinical features with top ten features listed above.
What is the implication, and what should change now?
• Clinical support tools to aid in timely medical and surgical management can reduce morbidity and mortality.
Introduction
Vertebral osteomyelitis and discitis (VOD) is an infection of the vertebrae and intervertebral discs that can be life-threatening, with effects on mortality and long-term morbidity (1). The incidence of VOD admissions in the U.S. has been estimated to be 4.8 per 100,000, with an average 9-day length of hospital stay. This results in a substantial cost burden (2). Notably, the number of cases has increased in recent years, a phenomenon which is likely attributed to a combination of factors including an aging population, intravenous drug use, and improved knowledge of diagnostics (2). Furthermore, despite significant advances in techniques to diagnose VOD and a greater understanding of infectious etiologies, the overall mortality rate remains high, up to 20%, even after appropriate clinical care (3).
Most VOD cases result from the hematogenous spread of primary infection to secondary sites in the vertebrae. Therefore, initial clinical sequelae are often dominated by additional manifestations in the primary infection site, such as the urinary tract, skin, and/or soft tissues (4). Delays in diagnosis can potentially result in suboptimal treatment and poor resource allocation (5). Currently, the initial treatment paradigm involves selecting one of the two therapies—medical management or a combination of medical and surgical interventions (6). To determine the optimal treatment course, spine surgeons must consider several factors, including, but not limited to, neurologic symptoms/deficits, spinal instability, disease presentation, and patient comorbidities/frailty (7-9). Consequently, there is a subjective component to these decisions, which results in some noticeable variability in management between clinicians.
In cases of delayed treatment, native vertebral osteomyelitis cases have a 25% mortality rate and can result in significant pain, neurologic deterioration, paraplegia, and deformity (6). VOD is associated with significant morbidity; around 33% of patients contract residual disability and about 15% of patients are left with permanent neurological damage. Delayed diagnosis further worsens clinical outcomes (10). The considerable variance in prognoses, coupled with a significant risk of unfavorable outcomes, underscores the essential need for the development of evidence-informed clinical support tools. These tools will prove invaluable for refined clinical decision-making and effective allocation of hospital resources, particularly for patients suffering from VOD (5,11-16).
Currently, there is no validated quantitative-based prognostic tool aiding early determination of the need for surgical intervention in a patient with VOD. In this study, machine learning (ML) models were employed to predict the need for surgical intervention in VOD patients using baseline metrics available at the initial presentation. We hypothesized that using a statistically robust ML algorithm would identify features that accurately prognosticate the need for spinal surgical intervention. We present this article in accordance with the STARD reporting checklist (available at https://jss.amegroups.com/article/view/10.21037/jss-23-111/rc).
Methods
Study data
This study included a retrospective cohort of 1,111 adult patients (18 years and older) from 2015–2019 who were diagnosed with VOD and underwent either medical management or surgical intervention. For inclusion in our prognostic models, patients had to have a VOD diagnosis assigned to their admission at some point during their stay. Pediatric patients (<18 years) and patients with tumors or traumatic etiology were excluded. ICD-10 diagnosis codes M46.2,3,4,5 were used to identify patients with concomitant VOD during their admission. This study was conducted in accordance with the Declaration of Helsinki (as revised in 2013). The use of this data for this study was approved by the Washington University Institutional Review Board, IRB ID Number: 202101050 and individual consent was waived.
Candidate predictors
Covariates of interest included age, sex, race, admit diagnosis, and the Elixhauser comorbidity index quantified by van Walraven’s score (17). The first-recorded heart rate, blood pressure, O2 saturation, serum comprehensive metabolic panel, serum complete blood count, serum lactate and blood cultures were also analyzed and used for feature selection.
Preprocessing and missing values
There were initially 535 features included in the study including but not limited to sex, age, race, Elixhauser comorbidity index, admit diagnosis, and first-recorded vital signs, laboratory values, and culture results. Any variables missing in more than 50% of cases were removed from consideration. Analysis of variance (ANOVA) i.e., ANOVA F-value between label/feature for classification tasks were then employed to select the top 50 features among the remaining variables, and missing data was replaced via mean imputation. Since there was a noticeable discrepancy between the sizes of the non-surgical and surgical populations, a balanced training dataset was generated via undersampling.
Primary outcome
Statistical analysis
All statistical analyses were performed using the Pandas and NumPy libraries (Python 3.10, Python Software Foundation). Descriptive statistics were used to assess baseline patient demographic and clinical characteristics. The chi-square test was used to assess categorical variables, while the unpaired two-tailed Student t-test was used to evaluate continuous variables (parametric). Every P value was two-sided. The cutoff for statistical significance was P<0.05.
Training, validation, and evaluation
A total of three classification algorithms (XGBoost, logistic regression, and K-nearest neighbors) were trained, tested, and validated to develop an interpretable model capable of predicting the likelihood of a spine surgical intervention in VOD patients during the same admission. The Scikit-learn library (Python 3.10, Python Software Foundation) was used to train the three classification algorithms. For the XGBoost ML model, hyperparameter tuning was accomplished via a grid search with cross-validation. Twenty percent of the dataset was set aside to test the models, and another twenty percent subset was used for validation. Both cross-validation resampling and bootstrapping were utilized during the validation step. Feature importance was assessed for the XGBoost model, which can directly output a feature importance score based on the mean decrease in Gini impurity. Sensitivity and specificity were calculated for each model and then used to build the receiver operating characteristic (ROC) curve. Model accuracy, area under the curve (AUC), sensitivity, and specificity were used to compare and evaluate model performance (Figure 1).
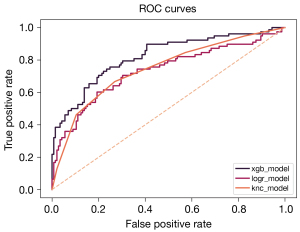
Results
Clinical characteristics
A total of 1,111 patients had a diagnosis of VOD, among which 70% (n=772) were managed medically, and 30% (n=339) underwent surgical intervention. With respect to cohort characteristics, age and sex did not significantly differ between the two groups; race, however, did differ substantially (P<0.0001), with the surgical group having a higher percentage of white patients (Table 1). Several of the top admit diagnoses were shared between the surgical and nonsurgical groups. However, an admit diagnosis of “intraspinal abscess and granuloma” was found more frequently in those patients who underwent a surgical intervention (Table 2).
Table 1
Model features (covariates) | Nonsurgical (N=772) | Surgical (N=339) |
---|---|---|
Age (years), mean (standard deviation) | 59.1 (16.1) | 59.4 (13.3) |
Sex, n (%) | ||
Male | 511 (66.2) | 211 (62.2) |
Female | 261 (33.8) | 128 (37.8) |
Race, n (%) | ||
Caucasian | 460 (59.6)* | 256 (75.5)* |
Black | 286 (37.0)* | 70 (20.6)* |
Other | 24 (3.1) | 10 (2.9) |
Asian | 2 (0.3) | 3 (0.9) |
BMI (kg/m2), mean (standard deviation) | 27.8 (8.4) | 28.5 (8.3) |
Length of stay (days), mean (standard deviation) | 10.1 (11.2)* | 21.4 (28.2)* |
Discharge disposition, n (%) | ||
Home | 467 (60.5)* | 173 (51.0)* |
Hospice facility | 18 (2.3) | 5 (1.5) |
Acute care hospital | 26 (3.4)* | 4 (1.2)* |
Nonacute care facility | 220 (28.5) | 138 (40.7) |
In-hospital death | 36 (4.66) | 16 (4.7) |
Miscellaneous/other | 5 (0.6) | 3 (0.9) |
Readmission, n (%) | ||
30-day | 160 (20.7) | 63 (18.6) |
60-day | 222 (28.8) | 92 (27.1) |
90-day | 267 (34.6) | 103 (30.4) |
Charlson score, mean (standard deviation) | 3.2 (2.7) | 3.2 (2.8) |
Elixhauser score (vanWalraven09), mean (standard deviation) | 10.6 (11.2)* | 12.1 (11.2)* |
Elixhauser score (Moore17), mean (standard deviation) | 10.8 (14.0)* | 12.8 (13.9)* |
Elixhauser comorbidities, n (%) | ||
Congestive heart failure | 168 (21.8) | 75 (22.1) |
Cardiac arrythmias | 190 (24.6) | 105 (31.0) |
Valvular disease | 86 (11.1) | 59 (17.4) |
Pulmonary circulation disorders | 51 (6.6) | 37 (10.9) |
Peripheral vascular disorders | 85 (11.0) | 41 (12.1) |
Hypertension | 474 (61.4) | 232 (68.4) |
Paralysis | 178 (23.1) | 64 (18.9) |
Other neurological disorders | 134 (17.4) | 63 (18.6) |
Chronic pulmonary disease | 151 (19.6) | 83 (24.5) |
Diabetes, uncomplicated | 71 (9.2) | 32 (9.4) |
Diabetes, complicated | 240 (31.1) | 101 (29.8) |
Hypothyroidism | 95 (12.3) | 42 (12.4) |
Renal failure | 180 (23.3) | 75 (22.1) |
Liver disease | 66 (8.5) | 40 (11.8) |
Peptic ulcer disease excluding bleeding | 10 (1.3) | 5 (1.5) |
AIDS/HIV | 5 (0.6) | 3 (0.9) |
Lymphoma | 11 (1.4) | 5 (1.5) |
Metastatic cancer | 41 (5.3) | 20 (5.9) |
Solid tumor without metastasis | 64 (8.3) | 31 (9.1) |
Rheumatoid arthritis/collagen vasculitides | 32 (4.1) | 22 (6.5) |
Coagulopathy | 83 (10.8) | 49 (14.5) |
Obesity | 108 (14.0) | 67 (19.8) |
Weight loss | 177 (22.9) | 94 (27.7) |
Fluid and electrolyte disorders | 270 (35.0) | 156 (46.0) |
Blood loss anemia | 5 (0.6) | 5 (1.5) |
Deficiency anemia | 52 (6.7) | 23 (6.8) |
Alcohol abuse | 34 (4.4) | 17 (5.0) |
Drug abuse | 109 (14.1) | 44 (13.0) |
Psychoses | 19 (2.5) | 14 (4.1) |
Depression | 178 (23.1) | 95 (28.0) |
*, indicates significance with P<0.05. AIDS/HIV, acquired immunodeficiency syndrome/human immunodeficiency virus.
Table 2
No. | Nonsurgical (N=772) | Surgical (N=339) |
---|---|---|
1 | Sepsis, unspecified organism (n=76, 9.8%) | Sepsis, unspecified organism (n=22, 6.5%) |
2 | Dorsalgia, unspecified (n=42, 5.4%) | Intraspinal abscess and granuloma (n=18, 5.3%) |
3 | Osteomyelitis of vertebra, lumbar region (n=34, 4.4%) | Osteomyelitis of vertebra, lumbar region (n=14, 4.1%) |
4 | Osteomyelitis of vertebra, sacral and sacrococcygeal region (n=31, 4.0%) | Dorsalgia, unspecified (n=12, 3.5%) |
5 | Fever, unspecified (n=29, 3.8%) | Osteomyelitis of vertebra, thoracic region (n=9, 2.7%) |
Gradient-boosted decision tree model prognosticating the need for surgical intervention
The best performing model, XGBoost, was trained on the 50 features selected by ANOVA F-value. These features each had less than 50% missing cases, and included a variety of demographic factors, such as race, as well as several clinical factors, such as admit diagnosis.
The top ten features with respect to predictive power were as follows (in descending order of importance): admit diagnosis of fever, negative culture, Staphylococcus aureus culture, partial pressure of arterial oxygen to fractional inspired oxygen ratio (PaO2:FiO2), admit diagnosis of intraspinal abscess and granuloma, admit diagnosis of sepsis, race, troponin I, acid-fast bacillus culture, and A-a gradient (Figure 2). Thus, these are likely important clinical predictors of a spine surgical intervention in the setting of VOD.
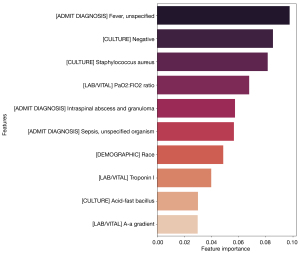
The model metrics for the model with greatest performance (XGBoost) were as follows: accuracy =0.7534, sensitivity =0.7436, specificity =0.7586, and AUC =0.8210 (Table 3).
Table 3
ML model | Accuracy | Sensitivity | Specificity | AUC |
---|---|---|---|---|
XGBoost | 0.7534 | 0.7436 | 0.7586 | 0.8210 |
Logistic regression | 0.6861 | 0.6538 | 0.7034 | 0.7325 |
K neighbors classifier | 0.7085 | 0.6667 | 0.7310 | 0.7456 |
ML, machine learning; AUC, area under the curve.
Discussion
Patients exhibiting neurological deficits due to epidural or paravertebral abscesses, those displaying spinal instability with potential or actual cord compression, as well as those for whom medical treatment has proven ineffective, constitute the clinical scenarios where spinal surgery is indicated. In such scenarios, the goal of surgical intervention encompasses decompressing the neural elements, securing culture specimens for targeted antibiotic therapy, and reinforcing the stability of the spinal column in instances of spinal instability. For a non-spine surgeon, the evaluation of who benefits from a spine surgical consultation is often delayed while awaiting an MRI to better characterize the extent of disease. In this study, three different ML models are presented that use readily available clinical data to predict the indication for a spine surgical intervention (during the same hospital stay) with modest performance. This tool could be used to assist non-surgeons in consulting spine surgeons early based on data readily acquired during a patient’s initial presentation to the emergency room or onto a medical floor. These results constitute the first evidence to date regarding the applicability of modern ML classifiers for predicting the indication for surgical intervention (during the same hospital stay) in patients with VOD (Figure 3). Additionally, this tool could improve resource allocation for patients with newly diagnosed VOD, as not all patients with VOD would benefit from an early spine surgical consultation. Moreover, our findings illuminate an imbalance in the administration of surgical treatment for VOD when comparing white patients to those from other ethnic backgrounds. The recognition of this latent disparity paves the way for a recalibration of clinical support tools, thereby mitigating the insidious sway of subconscious biases that may otherwise color the medical care provided to patients.
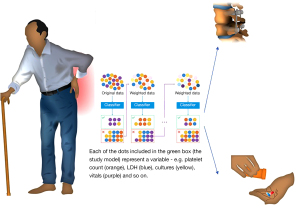
Predicting spine surgery in other spinal disease models
The ultimate objective of employing ML in spine surgery is to devise a “plug-and-play”, forward-looking model. The model would be able to ascertain optimal surgical candidates and predict clinical outcomes, relying solely on patient factors identified from the day of admission. Literature on the utilization of ML for preoperative prediction of intraoperative and postoperative outcomes in anterior lumbar spine surgery has already been explored (18), but studies on ML for the creation of decision-making trees for other spinal pathologies are limited. The literature has multiple ML studies pertaining to degenerative lumbar disc disease (DLDD) and spinal deformity. A majority of the studies are focused on predicting complications rather than assessing clinical outcomes associated with either surgical or nonsurgical management. The discernment of surgical candidates amongst nonoperative patients can alleviate the burden on the healthcare system, consequently mitigating overall cost of care or allocating costly care to patients most likely to have an optimal clinical outcome. Xie et al. created an Artificial Neural Network model to determine surgical candidacy for 387 DLDD patients. Of the initial 55 variables, eight variables were used for input into the algorithm. Their model had an AUC of 0.90, predictive accuracy of 0.92, and a Youden’s Index-determined sensitivity and specificity of 0.85 and 0.92, respectively. Severe canal stenosis had a normalized importance of beyond 90%, making it the most significant determinant in their model for surgical intervention (19). Wilson et al. created a deep U-Net ML model to determine which patients with lumbar stenosis would most likely require a laminectomy (20). The overall model had an AUC of 0.88, with an AUC of >0.70 at each level of lumbar stenosis. The sensitivity and specificity of the model were 0.81 and 0.85 (20), similar to the performance found in our models. These previous reports support the feasibility of implementing ML to model surgical decision-making.
Although the AUCs of the models we created were modest, the AUCs likely reflect that our models are not overfitting the dataset, which is a common issue with more flexible, less interpretable, and “blackbox” models, such as artificial neural networks or deep U-net models. Additionally, our model’s AUC performance will likely improve as we add patients to the dataset, and given that it is not overfitting the dataset, it would potentially perform better than more flexible when applied to clinical data from another institution on external validation.
Strengths and limitations
Our study utilized data from a comprehensive single-center database of patients with VOD that includes all flowsheet data, types of care support required during the index encounter [e.g., oxygen delivery, renal replacement therapy, need of intensive care unit (ICU) stay, transfusions], laboratory data, microbiological data, and operating room data. This registry offers higher quality data than those found in the traditional insurance claims databases and national registries which often have abstraction errors (21). As opposed to previous studies, which mainly employed models to identify risk factors for poor outcomes or complications after surgery, in this study, a model was developed with modest accuracy that predicts which patient will undergo a surgical intervention based on readily available clinical data at the time of admission.
This study has specific limitations. Primarily, the dataset is not specialized for spine surgery and is bereft of specific clinical factors that spine surgeons utilize to determine who stands to gain the most from such surgery. These important factors that are not available within the dataset include crucial radiographic findings like the severity of spinal cord compression and spinal instability, previous administration of targeted antibiotic therapy prior to surgery, and patient neurological status. Secondly, the ML models have only been internally validated, so future external validation is warranted to enhance the generalizability of the predictions. The findings of this study will serve as a foundation for future research to use predictive modeling and enhance the delivery of spine surgery for VOD. Lastly, the dataset used to train the ML models does not account for practice patterns and intrinsic selection biases that surgeons may have regarding the operative candidacy of a patient. As mentioned earlier, high-risk patients with significant medical comorbidities or poor social resources may be disproportionately managed nonsurgical. Consequently, this could introduce bias in the training of the model. Nevertheless, the intent behind these models would be to aid non-surgeon physicians in both emergency rooms and on medical floors in seeking consultation with a spine surgery service using clinical information that is commonly available early in a patient’s treatment course, even before an MRI is obtained. In other words, the clinical support tool utilizing the models from our study would not make definitive decisions about spine surgery, but rather provide a prompt and informed suggestion for consultation when pertinent.
Further research incorporating neurological status and pertinent imaging findings in a similar ML model will certainly add value to the model’s prediction accuracy, quality, and utility of results.
Conclusions
Based on the evaluation of three models, the XGBoost model predicts the indication for a spine surgical intervention (during the same hospital stay) based on several readily available patient demographic information and clinical features. This model was the most superior in terms of all performance metrics assessed. This tool may be a useful clinical decision-making support tool to optimize resource allocation and facilitate early consultation for spine surgery in VOD patients. However, further validation and impact studies are needed to fully realize the benefit of this prediction model.
Acknowledgments
We thank Dr. Andrew Michelson, Assistant Professor of Medicine at the Washington University in St. Louis for providing his institutional database. We additionally thank the Spine Division of the Department of Neurosurgery of the Washington University in St. Louis for helpful comments and edits.
This research has been presented at the 32nd Annual Meeting of The Society of Black Academic Surgeons (SBAS) and the 2023 Disorders of the Spine and Peripheral Nerve (DSPN) Spine Summit as a Kuntz Scholar Award-winning abstract.
Funding: None.
Footnote
Reporting Checklist: The authors have completed the STARD reporting checklist. Available at https://jss.amegroups.com/article/view/10.21037/jss-23-111/rc
Data Sharing Statement: Available at https://jss.amegroups.com/article/view/10.21037/jss-23-111/dss
Peer Review File: Available at https://jss.amegroups.com/article/view/10.21037/jss-23-111/prf
Conflicts of Interest: All authors have completed the ICMJE uniform disclosure form (available at https://jss.amegroups.com/article/view/10.21037/jss-23-111/coif). B.H.P. reports being a consultant for Cerapedics; N.A. reports receiving royalties from Thieme Medical Publishers and Springer International Publishing; C.A.M. reports being a consultant for Stryker, Augmedics, DePuy Synthes, and Kuros Biosciences; W.Z.R. reports serving as a consultant for Globus, DePuy Synthes, Nuvasive, Corelink, and Pacira and holding a patent with Acera outside the submitted work. The other authors have no conflicts of interest to declare.
Ethical Statement: The authors are accountable for all aspects of the work in ensuring that questions related to the accuracy or integrity of any part of the work are appropriately investigated and resolved. This study was conducted in accordance with the Declaration of Helsinki (as revised in 2013). The use of this data for this study was approved by the Washington University Institutional Review Board, IRB ID Number: 202101050 and individual consent was waived.
Open Access Statement: This is an Open Access article distributed in accordance with the Creative Commons Attribution-NonCommercial-NoDerivs 4.0 International License (CC BY-NC-ND 4.0), which permits the non-commercial replication and distribution of the article with the strict proviso that no changes or edits are made and the original work is properly cited (including links to both the formal publication through the relevant DOI and the license). See: https://creativecommons.org/licenses/by-nc-nd/4.0/.
References
- Zadran S, Pedersen PH, Eiskjær S. Vertebral Osteomyelitis: A Mortality Analysis Comparing Surgical and Conservative Management. Global Spine J 2020;10:456-63. [Crossref] [PubMed]
- Issa K, Diebo BG, Faloon M, et al. The Epidemiology of Vertebral Osteomyelitis in the United States From 1998 to 2013. Clin Spine Surg 2018;31:E102-8. [Crossref] [PubMed]
- Lener S, Hartmann S, Barbagallo GMV, et al. Management of spinal infection: a review of the literature. Acta Neurochir (Wien) 2018;160:487-96. [Crossref] [PubMed]
- Tay BK, Deckey J, Hu SS. Spinal infections. J Am Acad Orthop Surg 2002;10:188-97. [Crossref] [PubMed]
- Segreto FA, Beyer GA, Grieco P, et al. Vertebral Osteomyelitis: A Comparison of Associated Outcomes in Early Versus Delayed Surgical Treatment. Int J Spine Surg 2018;12:703-12. [Crossref] [PubMed]
- Berbari EF, Kanj SS, Kowalski TJ, et al. 2015 Infectious Diseases Society of America (IDSA) Clinical Practice Guidelines for the Diagnosis and Treatment of Native Vertebral Osteomyelitis in Adults. Clin Infect Dis 2015;61:e26-46. [Crossref] [PubMed]
- Connor DE Jr, Chittiboina P, Caldito G, et al. Comparison of operative and nonoperative management of spinal epidural abscess: a retrospective review of clinical and laboratory predictors of neurological outcome. J Neurosurg Spine 2013;19:119-27. [Crossref] [PubMed]
- Adogwa O, Karikari IO, Carr KR, et al. Spontaneous spinal epidural abscess in patients 50 years of age and older: a 15-year institutional perspective and review of the literature: clinical article. J Neurosurg Spine 2014;20:344-9. [Crossref] [PubMed]
- Arnold R, Rock C, Croft L, et al. Factors associated with treatment failure in vertebral osteomyelitis requiring spinal instrumentation. Antimicrob Agents Chemother 2014;58:880-4. [Crossref] [PubMed]
- Dholoo F, Sriramanarayanan A, Prasad SPK, et al. Spondylodiscitis-a cohort analysis of its identification and management. Int Orthop 2023;47:813-8. [Crossref] [PubMed]
- Ghobrial GM, Beygi S, Viereck MJ, et al. Timing in the surgical evacuation of spinal epidural abscesses. Neurosurg Focus 2014;37:E1. [Crossref] [PubMed]
- Zoabi Y, Kehat O, Lahav D, et al. Predicting bloodstream infection outcome using machine learning. Sci Rep 2021;11:20101. [Crossref] [PubMed]
- Choi SG, Lee EY, Lee OJ, et al. Prediction models for early diagnosis of actinomycotic osteomyelitis of the jaw using machine learning techniques: a preliminary study. BMC Oral Health 2022;22:164. [Crossref] [PubMed]
- Kim J, Ryu H, Kim SW, et al. Prediction of Recurrence in Pyogenic Vertebral Osteomyelitis by Artificial Neural Network Using Time-series Data of C-Reactive Protein: A Retrospective Cohort Study of 704 Patients. Spine (Phila Pa 1976) 2021;46:1207-17. [Crossref] [PubMed]
- Lalehzarian SP, Gowd AK, Liu JN. Machine learning in orthopaedic surgery. World J Orthop 2021;12:685-99. [Crossref] [PubMed]
- Tran NK, Albahra S, May L, et al. Evolving Applications of Artificial Intelligence and Machine Learning in Infectious Diseases Testing. Clin Chem 2021;68:125-33. [Crossref] [PubMed]
- van Walraven C, Austin PC, Jennings A, et al. A modification of the Elixhauser comorbidity measures into a point system for hospital death using administrative data. Med Care 2009;47:626-33. [Crossref] [PubMed]
- Karhade AV, Bongers MER, Groot OQ, et al. Development of machine learning and natural language processing algorithms for preoperative prediction and automated identification of intraoperative vascular injury in anterior lumbar spine surgery. Spine J 2021;21:1635-42. [Crossref] [PubMed]
- Xie N, Wilson PJ, Reddy R. Use of machine learning to model surgical decision-making in lumbar spine surgery. Eur Spine J 2022;31:2000-6. [Crossref] [PubMed]
- Wilson B, Gaonkar B, Yoo B, et al. Predicting Spinal Surgery Candidacy From Imaging Data Using Machine Learning. Neurosurgery 2021;89:116-21. [Crossref] [PubMed]
- Gliklich RE, Dreyer NA, Leavy MB. Data Collection and Quality Assurance. Registries for Evaluating Patient Outcomes: A User's Guide [Internet] 3rd edition. Agency for Healthcare Research and Quality (US); 2014.